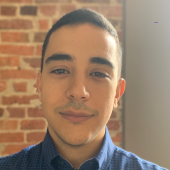
Hamza Salem
Former MSc Student
Hamza holds a BA in politics and computer programming from NYU. His research interests include using data to understand and predict political behaviour, mass mobilization, and social movements.
Predicting the outcome of the 2020 US Senate election using Wikipedia pageview data by Hamza Salem, Oxford Internet Institute MSc Alumnus and Dr Fabian Stephany, Researcher, Oxford Internet Institute.
In this blog we explain how we build a statistical model to predict US congressional elections using Wikipedia pageview data as a main indicator. We train our model on US House and Senate data from the 2016 and 2018 elections, and find it to be particularly useful in predicting the success of non-incumbent challenger campaigns. We use our model to forecast the outcomes of the 2020 US Senate election.
The prediction of electoral outcomes is not a new endeavour, and has remained a topic of public speculation in the United States since at least the 1880s. Traditionally, professional election forecasting in the United States has largely relied on direct polling to gain insight into public opinion. The availability of internet generated user data, however, has opened a new avenue allowing researchers to predict future human behaviour by using online interactions on social media platforms such as Twitter, and Facebook. Academic research on election prediction using such platforms has had mixed results, and has been largely met with criticism. Instead, we use Wikipedia pageviews as a measure of public information-seeking behaviour to predict the 2020 US Senate election.
In general, research utilising social media to gauge public opinion, often captures unique popularity on the platforms (rather than reflecting larger public interest in particular candidates), and overestimates the importance of discourse within a relatively small group of people. In order to avoid these pitfalls, we attempt to find online generated data that is both more representative of the general voting public, and which can more accurately demonstrate voter interest in a candidate, rather than a candidate’s general popularity.
In our recent paper, “Wikipedia: A Challenger’s Best Friend? Utilising Information-seeking Behaviour Patterns to Predict US Congressional Elections”, we argue that Wikipedia pageviews largely satisfy these criteria. We combine traditional and novel data sources, within the context of US Senate and House elections from 2016 to 2018, utilising both Wikipedia pageview data and campaign indicators. In general, our study indicates that Wikipedia data is particularly useful in predicting the success of challenger, rather than incumbent campaigns. While incumbents’ pages on Wikipedia are more likely to receive higher volumes of traffic, pageviews for challengers’ are significantly more predictive of success, especially when the candidate is perceived to be viable.
Our results also demonstrate that Wikipedia pageviews are most predictive in cases where candidates are underrepresented in public media outlets, and less predictive when they are well-represented. These findings highlight the importance of distinguishing between candidate popularity and voter interest when building predictive models of electoral outcomes.
Our model considers a relatively small number of components including (1) candidate’s proportion of Wikipedia pageviews in relation to others in a race, (2) state and district partisan stronghold values, (3) perceived viability (which we calculate using campaign fundraising), and (4) incumbency and challengership. We intentionally do not include any polling statistics.
To test our model, we forecast the results of the US 2020 Senate elections. As shown in Figure 1, of the 35 seats up for re-election, we predict 16 Democrats and 19 Republican candidates to win their respective races. We consider our results in the context of three major predictive models: the Cook Political Report, fivethirtyeight and the negative partnership model.
We find that our model aligns quite closely with the Cook Report’s overall predictions for all Solid, Likely, and Lean races. Where the Cook Report expects a Toss up – not predicting any winners – our model makes a number of predictions, which we present in Figure 1 (you can find an interactive version here). In most cases, our model aligns with fivethirtyeight and the negative partisanship model in overall race predictions, but differs in exact probability margins (see Figure 2).
Figure 1: State outcomes of our prediction for the 2020 Senate election.
Note: In the case of Iowa, Teresa Greenfield (D) was denied a Wikipedia page until late in the campaign (due to internal moderator conflict). We expect her campaign to be successful, nonetheless, considering the proportionality of voter interest in her campaign, which we gather from third-party sources including Google Trend data. This prediction remains in-line with fivethirtyeight’s polling aggregate.
We also found that our model is also useful in “jungle primaries” where multiple candidates from the same party run in the general election. In these cases, either fivethirtyeight or the Cook Report only expects a Republican or Democrat Win, however, our model is able to predict a singular winner (states shaded in light blue and red). These particular cases are Kelly Loeffler (R) in Georgia, (2) William Cassidy (R) in Louisiana, Ben Lujan (D) in New Mexico, Roger Marshall (R) in Kansas, and Bill Hagerty (R) in Tennessee. The exact probabilities for each of the 80 candidates included in our model are shown in Figure 2.