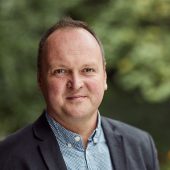
Dr Balazs Vedres
Former Senior Research Fellow
Dr Vedres is a sociologist and network scientist, investigating the network sources of creative success, and diversity and discrimination on online collaborative platforms.
Society generates copious amounts of data – we leave traces of social media posts, purchases, meetings, messages, and more behind. Even without being aware, we leave traces of our online surfing and physical mobility. The current pandemic has turned many of us from producers to consumers of data as well: we follow exponential curves, and R-rates of contagion reproduction to gauge the spread of the pandemic in various countries. We follow reports on an emerging economic crisis, tracing impact on various sectors of industry and government. We come to understand that news spreading on social media might be misleading, or junk, and that tracing data about the paths of spreading is our only hope to sort junk news from real news.
The pandemic crisis helps us appreciate that data is more than a simple summation of bits of information, but rather a lens onto complex relationships. Mobile phone location data from individuals can be aggregated to create alerts of crowding (and challenges for social distancing) at your supermarket. The R-rate of contagion itself is a complex interplay between biological properties of the virus (how infectious it was), and properties of our social networks (how many others we interact with). Reports mentioning firms from a sector of industry facing difficulties can become aggregated into an index anticipating economic crises by sector (see our recent report about the CoRisk-Index). We need to augment our senses with data to be able to cope with complex challenges.
The resilience of our societies against challenges that we are facing these days – the current pandemic, but also complex crises of climate, the economy, our political systems – will increasingly depend on how well our sensing capabilities develop. This sensing ability is greatly dependent on social data science: the flavour of data science that tackles social problems and is informed by social sciences.
Social data science is made possible by advances in computing power, and advances in machine learning algorithms that run on our ever faster computers. To illustrate such new possibilities, take the simple task of answering a yes/no question – predicting (using features of units of observations) if someone will become infected by the virus, that a firm will face bankruptcy, or that a piece of news is not genuine. Before tools from data science became available, we were using regression models for such a task. You might use a simple OLS (ordinary least squares) model (a linear probability model) that you could calculate on a page of grid paper. Someone today armed with machine learning tools would choose a random forest model – a model that is vastly more complex and involved than a regression model, as it aggregates a large number of decision trees, that each use a random subset of features and training data points. But why would one opt for a much more complicated (and computationally much more involved) method? The key again is social complexity. A regression model considers individual features by themselves only. Say you would want to predict who would catch the COVID-19 virus, based on demographic features. A regression model would treat age and gender (say, being male) independently, computing a coefficient for each. (You would probably see a positive coefficient for age, and a positive coefficient for a variable representing males.) The random forest model would enable you to explore the complex space of age and gender, identifying hotspots of infection with, say, men between 65 and 70, women aged 40-45, but also men aged 20-25, and women aged 30 to 35. In a regression model you might enter an interaction term for age and gender to account for a bit more complexity, but you would never be able to reach the complexity that a random forest model can explore.
While random forest models are fairly established, there are many exciting new areas of data science being employed to tackle social problems. Progress is constant in natural language processing (NLP): methods that take text as data, and help us find themes, sentiment, verbal violence at scale in large amount of text. Many of us at OII work on advancing NLP methods to understand fake news, online hate speech, gender discrimination, or effective political communication. There are exciting advances at the intersection of machine learning and network science, with methods taking entire network graphs as the input of learning, to predict outcomes for network communities based in the pattern of their connections.
A trust in data has its risks as well. One risk is a conceptual kind of social distancing – losing processes, action, understandings at the ground level of social actors from sight. It is tempting to jump to models and fancy machine learning solutions before developing intuitions. But taking a shortcut, and skimping on the groundwork of developing an intuition about how and why people do things carries grave risks. Our models might be built on spuriousness, overfitting, and ultimately might fail to make meaningful predictions. Even recognizing a meaningful problem in the first place is a work of trained intuition. Social data science is a way to enjoy the productive tension between exciting tools for the big picture, and enticing immersion in understanding where to deploy them.