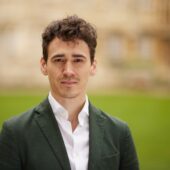
Dr Fabian Stephany
Departmental Research Lecturer
Fabian is a Departmental Research Lecturer in AI & Work at the Oxford Internet Institute.
The desire to know the future is as old as humankind. Often, people are particularly interested in forecasting political events. In the United States, for example, first attempts to forecast electoral outcomes can be dated back as far as to the 1880s [1]. Today, the rise of advanced statistics and large online data sources, has sparked new research endeavours in election forecasting. Computational and political scientists started to explore social media outlets, like Twitter or Facebook [2], to determine the outcomes of elections in advance. However, the use of “digital breadcrumbs” of social media data does come with limitations, too. As politicians actively promote their profiles on social media, this biased data do not necessarily reflect user interest and, ultimately, voting behaviour. Unbiased data is needed to predict election outcomes.
To detect the genuine interest of voters, we study pageview statistics from the online encyclopedia Wikipedia as an alternative data source for election predictions. In our recently published study, Wikipedia: A Challenger’s Best Friend? Utilising Information-seeking Behaviour Patterns to Predict US Congressional Elections [3], we showcase the relevance of so-called active information-seeking behaviour: Users who actively click on a politician’s Wikipedia page are likely to be interested in the candidate or her political agenda.
Indeed, with the example of the US Senate and House elections from 2016 to 2018, our study reveals that politicians who receive more pageviews prior to the election are more likely to win the race. Our predictions work best for new candidates with little prior visibility in the media. In numbers, for the case of challengers in open seat elections, Wikipedia pageview statistics improve conventional forecasting models by up to 21 percent points. Our best model has a prediction of accuracy of 85% vs. 64% for conventional forecasting models that do not consider Wikipedia data.
The desire to foresee the future prevails. People will always want to know what comes next. Big data allows us to observe human behaviour and make informed assumptions of future actions. But biases of gender, race, or geography often skew our view of the world [4], just like social media clicks might not reflect the true interest of voters. The less biased big data is, the more reliable are the forecasts we can derive from it – an important lesson when preventing the biases of today from shaping our image of tomorrow.
[1] Rhode, P. W., & Strumpf, K. S. (2004). Historical presidential betting markets. Journal of Economic Perspectives, 18(2), 127-141.
[2] Blank, G. (2016). The digital divide among Twitter users and its implications for social research. Social Science Computer Review, 35(6), 679–697. https://doi.org/10.1177/0894439316671698.
[3] Salem, H., & Stephany, F. (2021). Wikipedia: a challenger’s best friend? Utilizing information-seeking behaviour patterns to predict US congressional elections. Information, Communication & Society, 1-27 [open source].
[4] O’Neil, C. (2016). Weapons of math destruction: How big data increases inequality and threatens democracy. Crown.