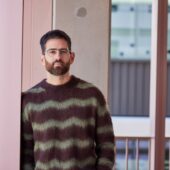
Ben Bariach
Former MSc Student
Ben is an alumnus of the Oxford Internet Institute and an expert in the safety and governance of artificial intelligence. He currently leads the Operational Ethics & Safety team at Google DeepMind.
With a proliferation of AI-generated images sweeping across the internet, OII alumnus Ben Bariach, Associate Professor and Senior Research Fellow Bernie Hogan, and Departmental Research Lecturer Dr Keegan McBride explore the benefits and risks of AI technologies for society.
As explicit AI-generated images of Taylor Swift spread across the social media platform X, issues of a person’s likeness and identity quickly came to the forefront of the public consciousness. AI likeness generation may make headlines when celebrities are involved, but it increasingly affects people outside of the public eye. The ability of AI systems to generate likeness is also not limited to the modality of imagery. Audio diffusion models can mimic a person’s voice, language models can emulate specific writing styles and imagery models may reproduce the unique oeuvre of known painters. What does it mean that someone’s likeness can be reproduced, and how will it impact society?
The term “likeness” refers to the recognisable characteristics of a person that sufficiently distinguishes them from others. AI systems are becoming increasingly adept at decoding and reproducing peoples’ likenesses. Just a year before the Taylor Swift incident drew attention to the issue of AI-generated likenesses, a more benign AI-generated image also went viral — Pope Francis wearing a puffy Balenciaga coat. The “Balenciaga Pope” was produced using the image generation model Midjourney, and showed that progress in generative AI goes beyond just large language models.
(AI-generated image of Pope Francis. Reddit, r/Midjourney)
Though these anecdotes are recent, concerns over the manipulation of an individual’s likeness go back centuries. Most recently, deepfake technologies enabled the creation of convincing videos representing synthetic depictions of people. However, recent advances in generative AI introduce conceptual and practical differences from these earlier “deepfake” technologies. Conceptually, newer generative AI models can “understand” and reproduce a person’s likeness rather than tamper with pre-existing features. Practically, newer generative AI models require less technical skills to use and produce outputs that are more realistic and harder to detect than ever before. Users who want to produce someone’s likeness no longer need to invest in developing drawing or photo editing skills, all they need is a keyboard.
Numerous benefits are emerging with these likeness generation advancements. The artist Grimes, for instance, encouraged the AI generation of her voice as long as she received royalties for its use. Users in pursuit of a job are already using image generation models to improve their headshots, and may easily edit photos of themselves or relatives. “Digitally resurrecting” musicians and movie stars are opening up new opportunities for the continuation of their legacy. We are still in the early stages of likeness generation, but it is likely that benefits will continue to emerge as generative AI becomes more popular.
Along with these benefits, however, risks emerge. Some harms associated with likeness generation are caused by misleading an audience into believing that a synthetic media being observed depicts a true representation of reality. One recent example of this was seen in the USA, where an AI-generated audio resembling Joe Biden’s voice was spread to New Hampshire voters, telling them not to vote. If the listeners knew the recording was fake, its harmful effect would mostly be mitigated. Various likeness generation harms rely on this misleading property, including misinformation, defamation, fraud, and the erosion of trust in digital media at a societal level. Harms that purport to represent a factual event can be effectively mitigated by revealing the AI-generated origin of an image with “watermarking” techniques. Once its true synthetic nature is revealed, users know that the alleged representation of likeness is not real. Consequently, the detection of synthetic likeness has become a field of inquiry in its own right.
Other likeness generation harms, however, do not depend on the viewer’s belief that what they see is true. In these cases, the harms don’t end when the image is revealed as fake. Consider the case of sexually explicit depictions of likeness, such as the Taylor Swift case. Refuting the veracity of the image does not sufficiently mitigate its harm. This is also the case for many other troubling categories of harms, including sexual content, child safety harms, toxic content, or representational harms. For such harms, revealing the synthetic provenance of the image is insufficient, and additional guardrails are needed.
The perceived truthfulness of representation is just one of the many nuanced features associated with likeness generation harms. Clarifying these features can help society address harms and identify the stakeholders with the most control over their mitigation. Another important feature is whether the harm occurred during the generation of the image or only with its distribution. For example, an AI-generated image of an observing Jewish or Muslim politician eating pork may not upset someone who asked to generate that image. However, if the image is distributed, it could be considered harmful misinformation. It is also important to consider when the likeness was introduced into the AI system, whether it was during the model training phase or through a subsequent user action such as uploading the image for editing or model fine-tuning.
Likeness generation is here, today, and so are its societal impacts. This year is slated to break records in the number of elections during a single year worldwide, just as likeness generation capabilities are booming, raising significant concerns about the threat of political misinformation. The recent Hollywood actors’ strike is a clear example where an industry-wide impact has already been felt due to the growing fear of the economic impact of likeness generation. The pressing implications of likeness generation underscore the need for efforts by researchers, industry, and regulators to ensure its safe development and deployment. Developing effective strategies to navigate the complexities of likeness generation is crucial for harnessing the societal benefits of this technology while mitigating its risks.
About the Author(s)
Ben Bariach (@benbariach) is an alumnus of the Oxford Internet Institute, University of Oxford. He is an AI safety and governance expert with experience spanning various tech roles at the intersection of technology and society. Ben also conducts AI governance and ethics research, with a focus on aligning AI with human and societal interests.
Prof. Bernie Hogan (@blurky) is an Associate Professor and Senior Research Fellow at the Oxford Internet Institute, University of Oxford. Bernie is a computational Social Scientist focusing on forward thinking methodological innovation and the role of design in social media. He is the Director of the OII Social Data Science programme, and takes a particular interest in philosophical issues related to AI and identity.
Dr. Keegan McBride (@KeeganMcB) is a lecturer in AI, Government, and Policy at the Oxford Internet Institute, University of Oxford. His research explores how new and emerging disruptive technologies are transforming our understanding of the state, government, and power.