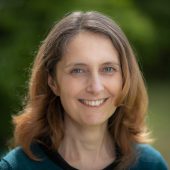
Professor Rebecca Eynon
Professor of Education, the Internet and Society
Rebecca Eynon's research focuses on learning and the Internet, and the links between digital and social exclusion.
The so-called “eternal spring” of AI has given new life to an old dream about supercharging human learning. From personalised teaching assistants to systems that “train” the brain directly, new tools and techniques enabled by AI seem to bring us ever closer to a technical solution to human learning.
Yet, this same “spring forward” that promises to accelerate human learning also creates worry that we must “robot proof” ourselves by learning lifelong and life-wide—even as notions of a “technical solution” to human learning import frames and discourses about the nature, role, and meaning of learning and education.
How, then, do we locate a critical assessment of artificial intelligence in lifelong learning?
Since the 1950s there has been a cyclical interest in the relationships between AI, Learning and Education, with a significant resurgence of attention over the past few years. Increased availability of large-scale data sets and expanding computational power have enabled computer and learning scientists within and outside the academy to re-engage with cognitivist learning theories and develop more advanced technological tools to facilitate personalised learning.
At the same time, a wider societal debate has emerged as AI becomes a part of everyday life, with hopes for a more economically prosperous future pitted against significant concerns about the potential negative impacts of AI on society such as the automation of many jobs. In this second area of debate, lifelong learning is typically promoted as a way to prepare society for an AI-enabled future and future-proof the workforce.
Clearly, AI is likely to have a role both in understanding and promoting learning for an individual, while at the same time having implications at a more societal level. However, the relationships between AI, learning, and education that are evident in these popular debates are so far relatively instrumental and narrow. For example:
Indeed, debating and identifying the wider democratic and personal outcomes of lifelong learning are an essential part of the debate at a time of significant social and technical change. Thus, to properly explore the potential of AI in lifelong learning a more holistic approach is required, that connects discussions of processes (i.e. in forms of personalisation) with discussions of outcomes (i.e. economic, democratic and personal). In doing this, we take a critical perspective.
Our approach in this project therefore:
Taking such an approach allows us to move away from a narrow range of questions about impacts (which risks being tied to ideas of the present) towards a broader and more nuanced discussion of what is or could be (that allows for the potential of alternative futures).
To provide the necessary foundations of such an approach we aim to do the following:
To assist with these two aims, and to encourage reflexivity about the value of AI, we are utilising a mix of social data science techniques along with more qualitative approaches to explore this area of work. We are also making the code open to others to use.
Attending to these multiple perspectives allow us to propose a way to conceptualise AI and lifelong learning to support a democratic and socially inclusive future.
We then use this as a framework to critically examine how AI is being used in practice to personalise lifelong learning and what the outcomes of such practices are for different groups and identify a series of recommendations for future work in this area.
We hope you’ll join us on this journey. Up next, we will shed more light on the tools, techniques, and datasets we have begun using in our research—as well as some preview of tools we are currently building.