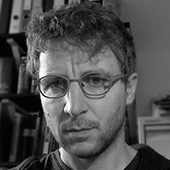
Private: Dr Patrick Allo
Former
patrick.allo@vub.ac.be
Critical evaluations of data science often combine ethical and epistemological criteria to explain how the practice of data science can fail to deliver on its promises to create better informed decision-making processes, or to lead to more efficient uses of available data. This has led an increased awareness of how a naive picture of the epistemological status of data and an uncritical understanding of the reliability of code can lead to unrealistic expectations, and may even create a context in which data-intensive knowledge practices become immune to certain forms of criticism or contestation.
When compared to the fervour with which the “myth of raw data” and the virtues of mechanical objectivity have been exposed by various scholars and commentators, the mathematical foundations of data science have not been subjected to the same level of scrutiny. This is surprising if we take into account that mathematical innovations, under the guise of algorithm design, are one of the driving forces behind the rise of data science, and that the rise of statistical reasoning has historically been associated with the desire to measure, quantify, and control the social. It is also unwarranted because the epistemological status of data science can at least partially be retraced to the peculiar nature of mathematical knowledge (often described as infallible, absolute, eternal, etc.). When the availability of mathematical theorems can be used to explain why (and under which circumstances) certain forms of inference are reliable, moderate versions of such views are warranted. This connection between the reliability of mathematics and the reliability of data-science becomes, however, vacuous, when it simply refers to the commonplace idea of “trust in numbers,” and the added value of mathematics is almost indistinguishable from the initial value of the data (measuring and counting is where mathematics and data come together). Such vacuous connections become problematic when they are part of the reigning rhetoric that is used to defend data-intensive practices, turn into forms of mathwashing, and can serve as a shield to protect questionable practices from legitimate objections. Here, mathematics no longer plays its emancipatory role, exemplified by the standard of mathematical proof that forces one to make things explicit and express ideas and arguments in an unambiguous language. Instead, mathematics behaves as a gate-keeper: it is used to attribute certainty where none is to be found, and to deny access to the uninitiated.
These concerns, as well as the simplistic views on mathematics it relies on, formed the basis for a panel-session organised at the latest conference of the Society for Philosophy and Technology, and intended to bring together different perspectives on the epistemic and societal role of mathematics in its relation to data-science and the data-revolution. The overarching perspective was that of actual mathematical practices (how mathematics is done, evaluated, and applied), and replaces the traditional focus on secure foundations with a more realistic understanding of mathematics as a human, and increasingly often computer-mediated practice. The interventions ranged from philosophical perspectives on the nature of pure and applied mathematics, to questions of methodology in statistical modelling, and the place of mathematics and statistics in education.
During her intervention, Karen François relied on research in mathematics and statistic education, and on her experience with the organisation of a graduate training-network for methodology and statistics. She reviewed the recent history of the concept of statistical literacy, and highlighted how it shifted from the basic need to understand and be able to apply statistical techniques, to a broader conception that is explicitly connected with ethical and political aspects, and includes the skills citizens need to interpret and criticise statistical information and statistical reasoning. Interestingly, the more general concept of mathematical literacy—as for instance used by the OECD in the context of PISA—underwent a similar evolution, and is now customarily related to the needs of constructive, concerned, and reflective citizens. These parallel evolutions mirror the current gap between purely technical and socio-political characterisations of Big Data.
Christian Hennig, a statistician and expert in cluster-analysis, developed a critical account of the role of model-assumptions in statistics. After drawing attention to the fact that most model-assumptions rest on (strictly speaking false) idealisations and explaining the conceptual challenges involved with the idea of checking model-assumptions (the goodness of fit paradox), he advanced an alternative understanding of mathematical modelling that focuses less on the truth of a model (and its assumptions), but instead explains how the specific virtues of using mathematical techniques further the scientific goal of establishing agreement in open exchange. He concluded by arguing for the value of evaluating the usefulness of a model by asking whether data could lead the methods based on this model astray, and presented this as the only feasible way of dealing with model-assumptions.
With the presentation of Johannes Lenhard, entitled “Ignoring the Grammar of Things,” the epistemology of Big Data came into focus, and was approached via a comparison of the role of mathematics in the sciences. By describing how Galileo’s early mathematisation of physics put forward the goal of making nature intelligible by revealing the mathematical structure of natural laws, and juxtaposing this ideal with Chomsky’s goal of making explicit the structural features of language, Lenhard characterised the traditional role of mathematics in the sciences as the formulation of the basic principles of composition of natural and human phenomena. This role was then contrasted with how currently popular machine learning techniques, like deep neural networks, depart from the traditional ideal of acquiring knowledge of basic principles to gain insight. Indeed, machine learning based on a universal architecture appears to ignore the (familiar) grammar of things, and adopts an agnostic mode to learning. Despite being enabled by mathematics, these forms of learning therefore go against the initial promise, still associated with how Galileo used geometry to make physics intelligible for humans, of the value mathematics within the sciences.
The presentation of Jean Paul Van Bendegem started from what is at first blush a typical question for the philosophy of mathematics, namely “what is the nature of mathematics and of mathematical knowledge?”. What he showed is that the ideal of a pure mathematics was an idea that had to be invented, and that, because it often remains unchallenged, can misrepresent mathematical theories and practices as epistemologically innocent. From the commonplace that most of pure mathematics is useless, and even doesn’t have to be useful to be valuable, it is only a small step to describe it as neutral, disinterested, and value-free. With this in mind, it is not hard to see how an unchallenged philosophy of pure mathematics can become an ally of the rhetorics of Big Data and its love of non-parametric methods and assumption-free science. Moving away from this broadly Platonistic conception of mathematics, and adopting a more constructivist and application-aware conception of mathematics that places mixed and hybrid mathematics at the centre, and recognises that counting and measuring practices arise becomes something is valuable enough to be quantified in the first place, is essential if we want to avoid that an unrealistic philosophy of mathematics reinforces the dominant ideology and unrealistic epistemologies that are used to promote the virtues and benefits of Big Data.