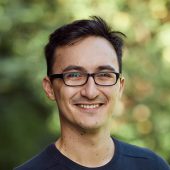
Dr Chico Camargo
Research Associate
Dr Chico Camargo is an interdisciplinary researcher who develops and applies computational tools to study complex systems, collective human behaviour, public opinion dynamics and culture change.
Understanding and forecasting traffic has long been a problem for policymakers. Road networks in and around our towns and cities are increasingly congested, yet traffic data is expensive to obtain. This makes it hard for town planners – with limited resources – to make informed decisions about where to build new developments.
In our new paper, ‘Estimating Traffic Disruption Patterns with Volunteered Geographic Information’, co-authored by Dr Chico Q Camargo, Dr Jonathan Bright, Dr Graham McNeill and Dr Scott Hale, together with Sridhar Raman from the Oxfordshire County Council, and published by leading journal Nature Scientific Reports, we develop new data science methodology to online mapping data to better understand traffic patterns.
In this new study we specifically analyse data from OpenStreetMap (OSM), to understand what types of land use are associated with traffic jams and increased traffic volume around points of interest within Oxfordshire. We use OSM features to predict traffic disruptions and traffic volume at 6,500 places in the road network within 112 regions of Oxfordshire.
Our analysis reveals some general trends in relation to traffic disruptions and traffic volume. Firstly, that commercial areas are most associated with high traffic, whereas the opposite is true in industrial areas. Secondly, we found little difference in the level of traffic disruption on weekdays compared to weekends. Thirdly, the kind of land use can be used as good predictors of the level of traffic disruption in different parts of Oxfordshire.
In terms of the impact of land use on traffic flow, our analysis shows that commercial land use is the biggest highest predictor of traffic volume, such as fast food outlets and cafes, followed by recreational and institutional land use, whereas industrial land use was the lowest predictor of traffic volume. In terms of traffic disruptions, residential land use and recreational land use was the most likely predictor of traffic disruptions, whereas in contrast, institutional land use such as University buildings and parks least likely predictor of traffic disruption.
Our findings demonstrate how using online mapping data incorporating detailed breakdowns of land use categories can be used in new ways to estimate volume and traffic disruption patterns. For example, the successful application of this data and methods could help policymakers and planners to understand more about how the impact of a new school on the number of traffic disruptions in its area should be comparable to the impact of a new retail store, helping policymakers and planners make informed decisions about urban planning.
Our study also suggests some promising areas for future research. One such area worth exploring would be to take advantage of the constantly evolving nature of OpenStreetMap to track the emergence of new physical features and relate those to changes in traffic conditions. Another would be to combine these with other sources of observational data, such as licensing applications, planning permissions, and building regulations, to see if these can build on the baseline model we have constructed. We would also consider extending our study to other countries and contexts adding an international dimension.
Ultimately, in the longer term, as our ability to understand and explain traffic patterns improves so will the ability of policymakers to effectively design urban transport systems that serve the needs of their citizens.